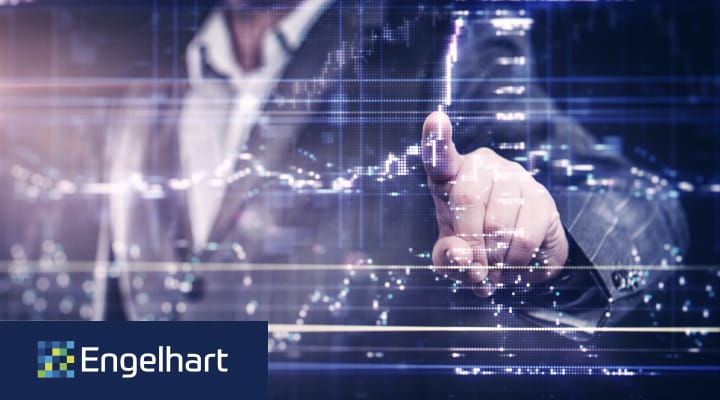
Interview with Mark Keenan, our Head of Commodity Strategy and Research
The following is an interview with Mark Keenan, the Head of Commodity Strategy and Research at Engelhart.
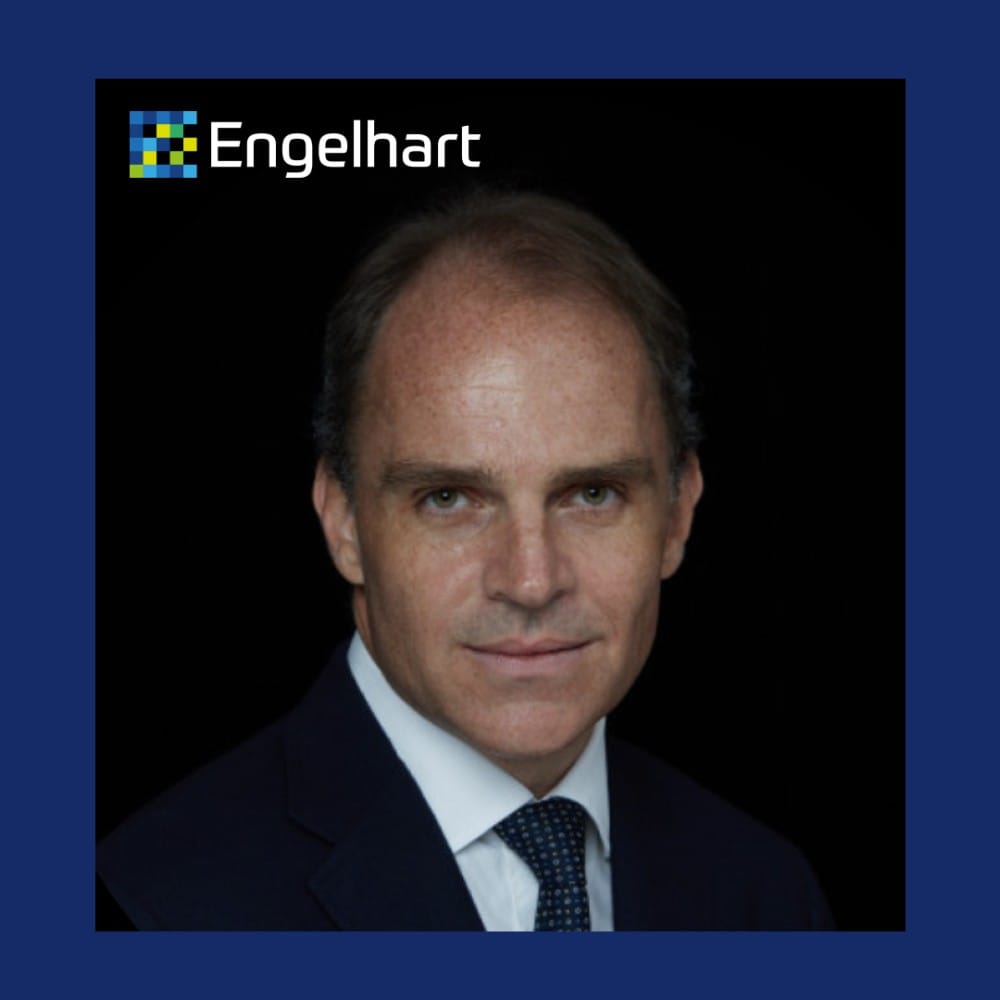
Mark has been at the forefront of quantitative research and strategy in commodities for the last 25 years. That’s why we wanted to get his reflections on how these quant strategies have developed in that time and what the future might hold.
Mark has also published two books on the subject.
You can buy the second book – “Advanced Positioning, Flow, and Sentiment Analysis in Commodity Markets: Bridging Fundamental and Technical Analysis”
We hope you find the interview interesting. Please share it with your networks and let us know your thoughts in the comments below.
Could you tell us about your career journey from university to your current role as Head of Commodity Strategy and Research at Engelhart?
I studied biochemistry at Oxford and was particularly focused on the quantitative side of the subject, which at the time involved sequencing of the human genome, essentially looking for patterns in vast amounts of data. It felt like a natural step after university to look towards a career in commodities, not only being able to use my interest in chemistry but also those quantitative skills, which were increasingly transferrable at the time, as commodities markets were transforming in terms of the available data.
So, after university, I entered the commodities sector and have spent about 25 years working in almost every area of financial commodities, from broking and sales to trading and research.
My career in commodities has had three main phases. The first was roughly ten years, focusing on price analytics and different ways of thinking about trend and price behaviour — a period heavily grounded in technical analysis.
“Commodities markets were transforming in terms of the available data.”
The second phase coincided with the proliferation of data, leading to a more quantitative approach to fundamental analysis. This allowed us to use the same framework, discipline and rigour that a technical researcher applies to price dynamics to fundamental elements like production, inventory, imports, and exports. Essentially, the birth of “quantamental analysis.”
Then, the third phase, which probably began ten years ago, was adapting to this data availability, which was now so prolific that finding a competitive edge became increasingly more challenging. That’s when a more sophisticated approach to areas like positioning, flow and sentiment analysis became vital to understanding the commodities markets we’re operating in.
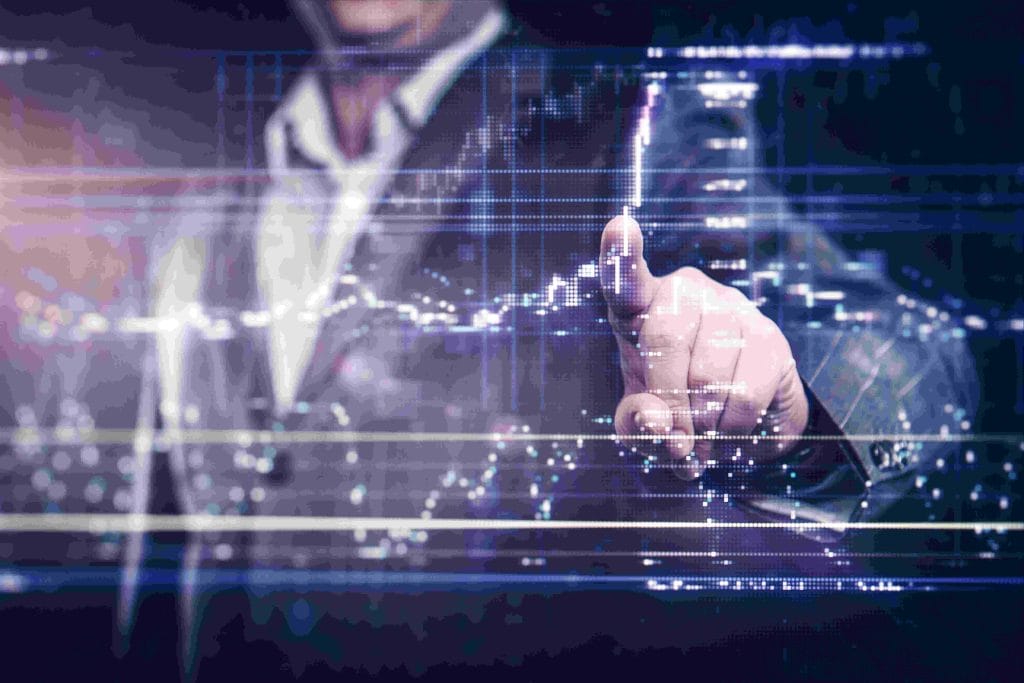
How exciting was it to be working in the industry when there was this sudden proliferation of the data available to commodities traders?
It was a huge opportunity, especially if you were thinking about developing a more quantitative approach, as I was. From the mid-2000s onwards, you suddenly had access to satellite data, tracking data, and many other interesting datasets. For example, we could now measure the height of storage tanks from space using shadow length. We could also look at the temperature of oil pipelines in the desert to indicate the speed of crude oil transmission inside them and we could measure the chlorophyll density of emerging crops from space to gauge potential yields.
Until then, quantitative innovation was mostly happening in commodities on the technical analysis side. However, for fundamentals, it was still a case of using a balance sheet and trying to calculate them yourself. This period was a big leap forward in the quality of research and trading that was possible. We could now blend price signals and fundamental signals in the same framework for the first time.
“People were constantly experimenting with new strategies, and it was very exciting”
Technology also accelerated rapidly in that period. I remember a software package I managed to get called Omega Trade Station, in which you could program a strategy of almost any order of complexity. Then you could press a button, and it would back-test it for you and generate your P&L, your risk and so on. At the time, it was very advanced.
Those software developments, alongside the beginning of the internet and the growth of information sharing on forums and chat rooms, meant that it all took off like wildfire. People were constantly experimenting with new strategies, and it was very exciting.
However, it also demonstrated an important point, which is that it’s easy to get carried away thinking you can find the holy grail of a strategy that will give you consistent returns. But the longer you do it, and the better you become at it, the more you realise it’s an ever-shifting landscape. It’s more important to be flexible and agile and constantly innovate and test. Those are the lessons I’ve taken from my experience and in observing the most successful funds and investors.
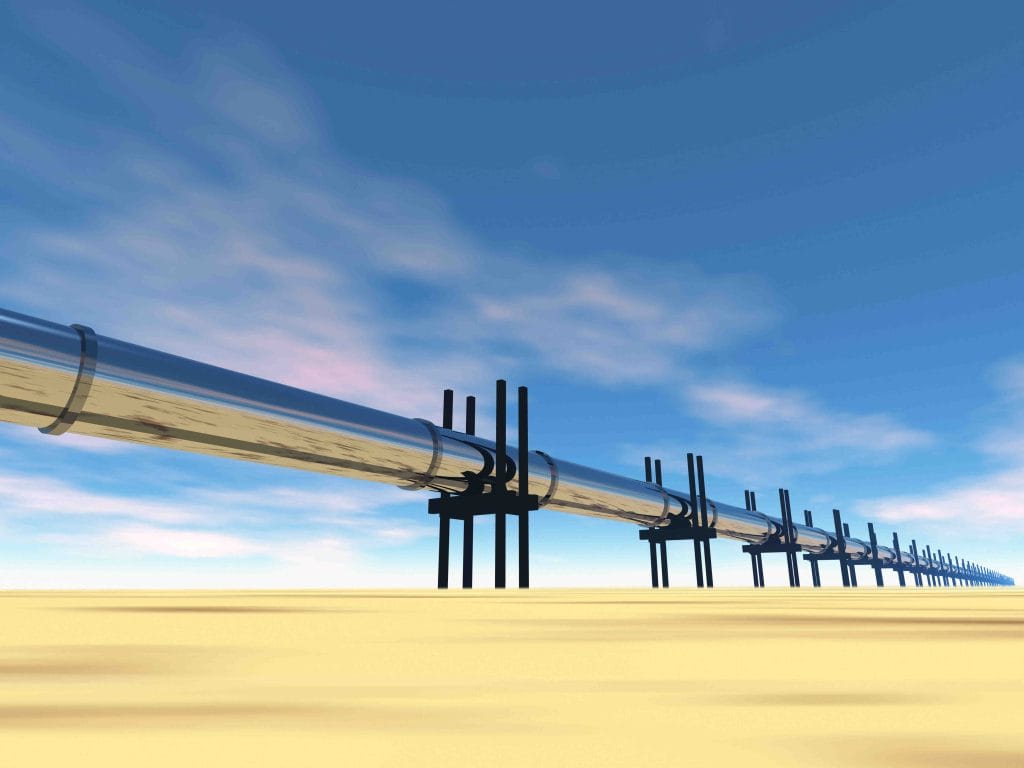
The third phase you mentioned also coincided with the increasing financialisation of commodities?
We’ve seen that trend emerge over the last decade or so. Commodities are now playing a much more significant role in investment portfolios, and these investors probably don’t know much about the underlying commodity.
They’re using them to enhance diversification and protect their portfolio against risks like inflation. I frequently talk to fundamental traders who say their markets are often completely decoupled from the fundamentals. They’re now just following the macro risk, and they’re just being rolled over by the financial flow. In some agricultural commodities, for example, more than 50% of the market is financial index exposure.
At Engelhart, our trading desks are mostly fundamentally orientated, and I always believe that fundamentals will eventually win out. However, using quantitative research tools is essential because it allows our traders to understand every bend in the road that takes you to that endpoint; it helps them understand macro effects, positioning risks and flows that might derail their trade or put their positions underwater.
“I frequently talk to fundamental traders who say their markets are often completely decoupled from the fundamentals.”
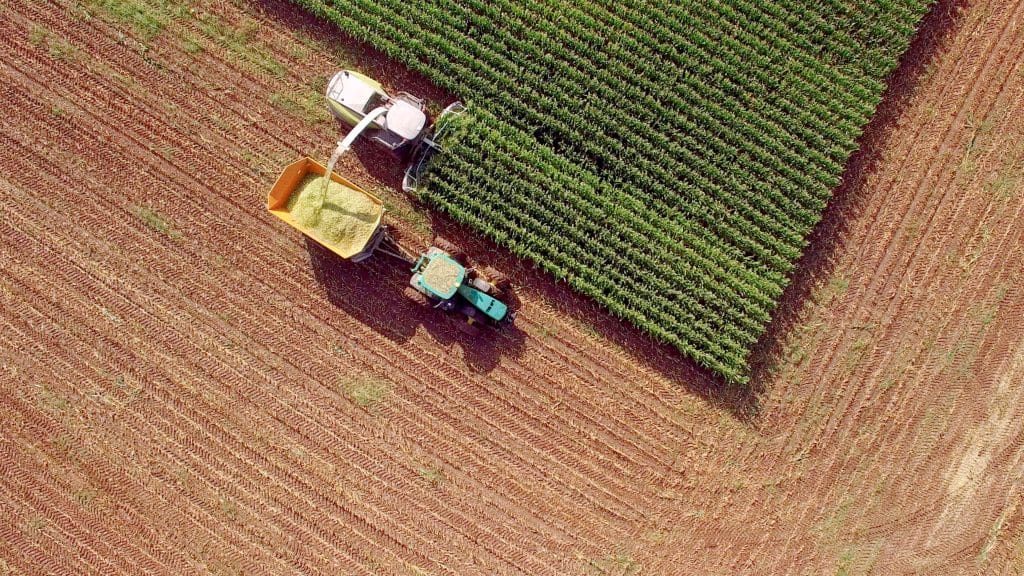
We’ve seen a rise in systematic trading strategies using quantitative research and algorithms in commodities markets. How do you assess their evolution and adoption in commodities?
In many ways, intraday algorithmic trading has become extremely dominant in some commodities markets. Looking at the TTF (Dutch natural gas futures), it appears that many of the largest traders of TTF in Europe now are mostly algorithmic intraday trend market participants as distinct from your more traditional market participants who would be hedging their TTF requirements, for example.
The growth in technology and the proliferation of algorithmic intraday trading activity have gone hand in hand. Similarly, the growth in QIS (quantitative investment strategies) or risk premia strategies has become another area of significant growth — by our calculations; there’s about $55-$60 billion invested in risk premia strategies.
These strategies are very quantitatively driven. They seek to monetise factors in commodity markets like carry, congestion, trend and value, and they are an effective response to the increasing financialisation we’ve seen.
By understanding these strategies, we are better placed to help our traders navigate these flows that can cloud and confuse the fundamentals.
Why do you think these strategies are so effective for trading commodities?
This idea works so well in commodities because there is often a strong linkage between commodity prices and changes in the fundamental data. This is underpinned by the fact that commodities are tangible goods, whose physical price ultimately needs to converge to traded derivative’s price.
Furthermore, as changes in commodity fundamentals tend to be serially correlated, meaning that they have a degree of persistence over time, hedging and trading programs help to align these changes with price over time. This underlying inertia makes many of these strategies so effective in commodities.
So, for example, if you have a movement of metal out of a warehouse or shipment of grain from a port, it takes time to move due to logistical constraints. If you look at LME(London Metal Exchange) inventory data or export shipment numbers, those time series are particularly serially correlated. Of course, you can’t trade them directly, but you can trade the price response to that time series.
“This idea works so well in commodities because there is often a strong linkage between commodity prices and changes in the fundamental data.”
Well-built trend algorithms and certain factor-driven strategies in commodities have historically generated reliable returns for decades. That success comes in part from the fact that commodities are tangible goods, they follow classic storage economics, they can only be produced and transported so quickly, and there is, therefore, often a lag in how quickly prices are able to respond to changes in supply and demand.
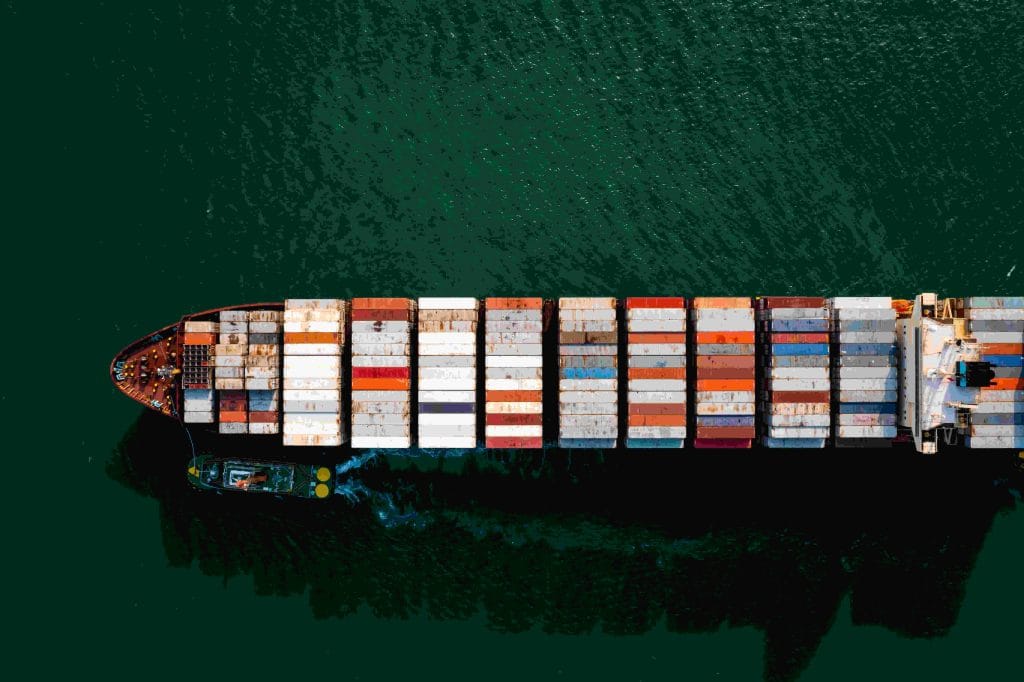
How effective are those strategies in the smaller, less financialised commodities markets?
They work well in those markets. We’ve seen the best returns recently from markets like cocoa, orange juice, and coffee, where the relative lack of liquidity helps because it’s difficult for a whole order to be executed instantaneously. If you’re using these markets to hedge, that’s done over time, and the prices will respond.
This gives the trend algorithm something to work with. It’s the opposite of what we see in equities when a company releases its news, and the price adapts instantaneously to some degree.
The benchmark commodities, broadly defined as those in the Bloomberg Commodities Index, have performed pretty badly on a trend basis recently. One of the reasons for that may be their increased financialisation and the fact that the market participants are now so diverse and their trading objectives so varied. Those non-benchmark markets I’ve mentioned have offered persistent returns because those prices tend to run; there’s more overshoot and fewer market participants, with fewer algorithms trimming the gains and cannibalising the quality of the price returns.
“We’ve seen the best returns recently from markets like cocoa, orange juice, and coffee.”
The challenge now is that more participants are entering these markets looking for these historical returns from these alternative markets, like carbon, TTF and UK natural gas, so we spend a great deal of time researching and identifying any changes in character to these markets to support our traders maintain their edge.
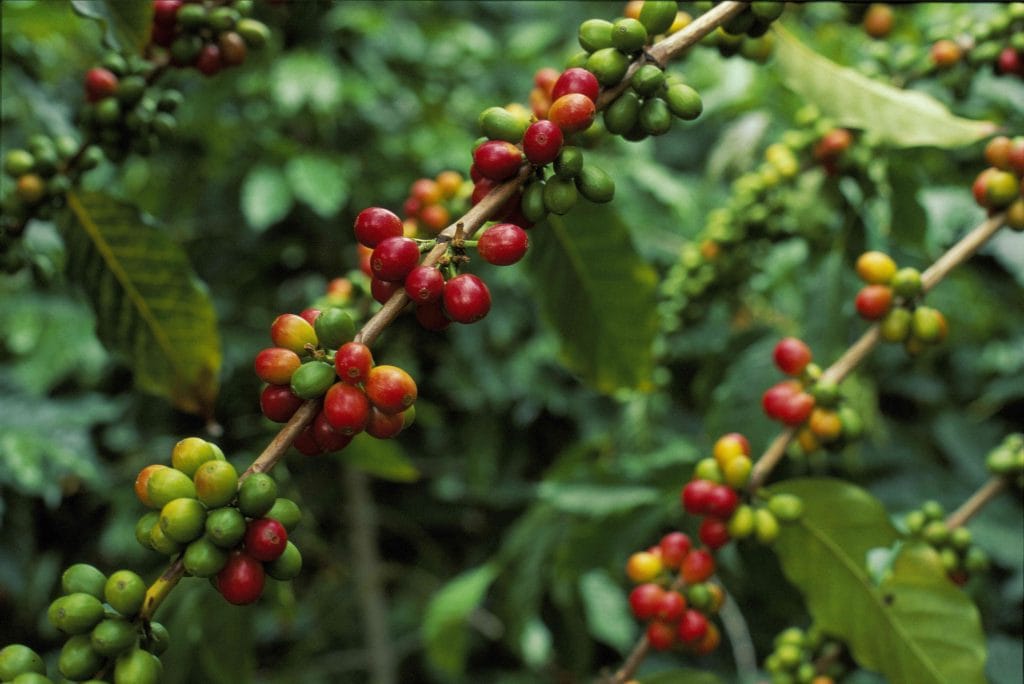
How important are risk premia strategies to your work? And what excites you about the opportunities in that space?
QIS, or risk premia strategy, is a real focus for Engelhart. My team and I spend 75% of our time on research and then 25% managing the QIS portfolio, where we’re constantly innovating and building new products to maintain an edge in that space.
It’s an area that’s developing quickly, and it’s exciting to work in it. We can now execute more and more complicated strategies on a cost-effective basis. The complexity of the portfolio we run has grown with the development of better execution algorithms, more cost-effective ways of running risk, and better ways of handling liquidity.
That’s allowed a much deeper granularity when looking at certain factors. We can build portfolios that can be very focused and deliver exactly what our objective is.
How does your team operate within Engelhart?
Our mandate as a team is to work across the whole firm, with all traders in all markets and provide them with research, data analytics and macroeconomic insights. What will this news mean for the price of copper? What does this data out of China mean for commodities?
We aim to give the trading desks as much added value as possible. Most teams will have their fundamental analysts doing their supply-demand balances. They don’t typically have someone dedicated to looking at all the flow, positioning, sentiment analytics, macro analysis, etc. So, that’s where we can support them.
The traders will always have their fundamental view, either bullish or bearish, and we provide the data that can help their decision-making. We don’t want our traders to be blindsided by anything that gets in the way of a core fundamental soybean view or their view of natural gas.
Most of the work is very creative. We’re always building new models that respond to the changing markets, work to maintain their relevance and ensure they are easy for our traders to understand and use effectively. For example, much of our recent work for example has focussed on specific types of pain metrics that we do in real-time across the curve. This helps traders anticipate potential flows when thresholds become extreme.
“We don’t want our traders to be blindsided by anything that gets in the way of a core fundamental soybean view or their view of natural gas.”
We’ve also done a lot in the last year looking at the impact of interest rates on commodities. Have they been bullish or bearish? How do rates change the structure of commodity curves? What does it mean for flows? What does it mean for the opportunity sets? Are those market participants who were high-frequency commodity traders now thinking, “Let’s just put the money in the bank and earn our 5%”?
The interesting thing about this space is that there are constantly new dynamics that we have to contend with. Then, we link those back into the commodity world to see what they might mean for price behaviour.
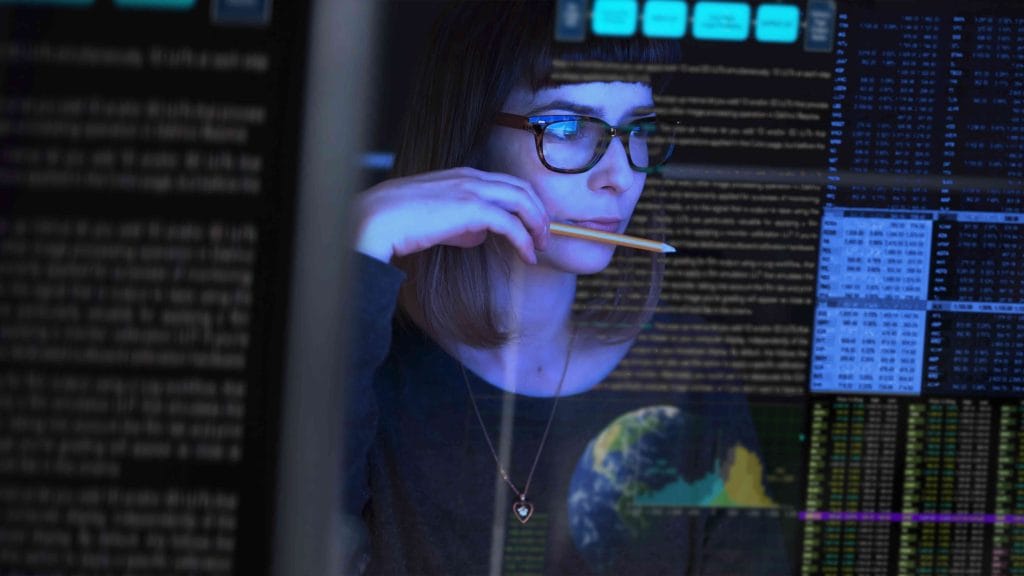
What are you looking for when you’re hiring a new researcher?
Creativity is always essential, alongside that entrepreneurial mindset and the ability to find solutions to problems independently. We’re a small team, so flexibility and agility are also vital.
It’s essential to always be on the front foot, always thinking ahead and understanding that the landscape changes, and then asking yourself how you adapt to those changes.
“It’s challenging yet meaningful work, and it’s kept me going for 25 years!”
From a practical standpoint, we do everything in Python. Hence, those coding skills are essential, and you have to be able to build models quickly and accurately.
I would tell anyone thinking about a career in the quantitative side of commodities that it’s a fascinating industry. You can build strategies based on how commodities markets have worked for the last 5000 years. In many ways, we’re honouring that historical relationship between scarcity and price and modelling ways to understand that better. It’s challenging yet meaningful work, and it’s kept me going for 25 years!